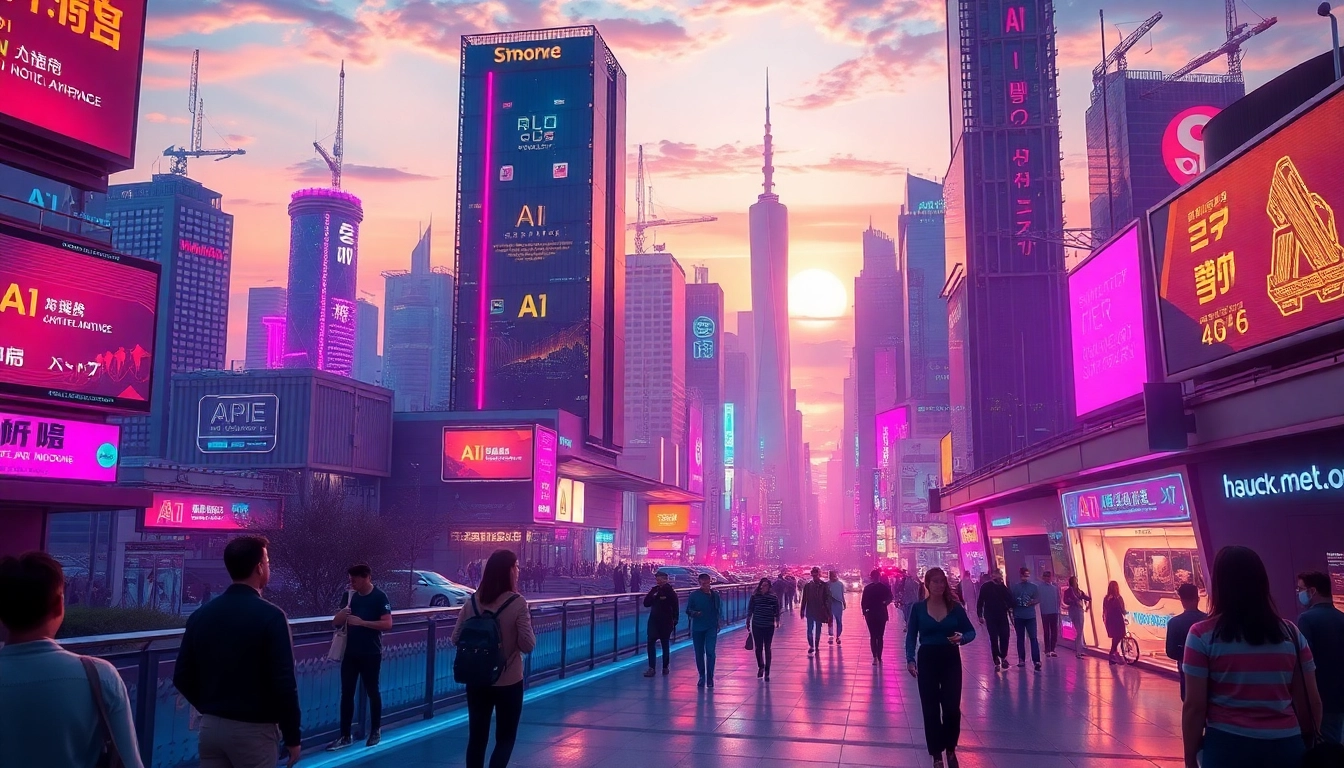
What is Agentic Human AI?
As the digital landscape evolves, so too do the technologies that define our interactions and capabilities. One of the most transformative advancements in artificial intelligence is the concept of Agentic Human AI. This innovation is characterized by AI systems designed to act autonomously to achieve goals while requiring minimal human intervention. It represents a shift from traditional AI paradigms, focusing not just on executing tasks but also on making decisions, adapting to circumstances, and enhancing productivity across various sectors.
Defining Agentic AI and Its Capabilities
Agentic AI, often referred to as autonomous AI, encompasses systems capable of reasoning, problem-solving, and decision-making without necessitating continuous human oversight. These systems operate based on structured programming combined with advanced machine learning algorithms, allowing them to learn from experiences and optimize their performance over time.
The capabilities of agentic AI can be broken down into several key areas:
- Autonomy: The ability to function independently, making decisions based on data inputs and contextual analysis.
- Adaptability: The capacity to modify responses and strategies in real-time, responding effectively to changes in the environment or objectives.
- Complex Problem-Solving: Utilizing advanced algorithms that can interpret complex data and devise solutions or strategies that are not explicitly programmed.
- Contextual Awareness: Recognizing and understanding the context surrounding a decision to enhance relevance and effectiveness.
Historical Background and Evolution
The roots of agentic AI can be traced back to the early developments in artificial intelligence, where initial models aimed to mimic basic human decision-making processes. Over decades, advancements in computational power, data analysis techniques, and machine learning algorithms have paved the way for more sophisticated AI systems.
Some pivotal milestones in this evolution include:
- The development of expert systems in the 1970s and 1980s, which aimed at mimicking human experts in specific fields.
- The introduction of machine learning in the 1990s, allowing systems to improve through experience.
- The rise of deep learning and neural networks in the 2010s, which significantly enhanced the capability of AI to process and analyze large datasets.
- Current advancements in reinforcement learning and self-supervised learning, which are crucial for creating truly independent agentic AI systems.
Key Characteristics of Agentic AI
To differentiate agentic AI from traditional AI systems, several key characteristics must be highlighted:
- Self-Regulation: Agentic AI can monitor its performance and make adjustments when necessary, ensuring optimal operation over time.
- Goal-Orientation: These systems are designed to pursue specific objectives, making them inherently purposeful in action.
- Interactive Learning: Through interaction with users and other systems, agentic AI can learn and adapt its strategies for improved outcomes.
- Comprehension of Past Experiences: Agentic AI utilizes historical data to inform future decisions, allowing for a feedback loop that enhances decision accuracy over time.
The Mechanisms Behind Agentic AI
How Decision-Making Works
The decision-making capabilities of agentic AI are fundamentally rooted in algorithms that process vast amounts of data to identify patterns, predict outcomes, and recommend actions. Here’s a brief overview of these mechanisms:
- Data Input: Agentic AI systems receive inputs from various sources, including sensory data, user interactions, and environmental factors.
- Data Processing: Using machine learning algorithms, the system analyzes the data to identify trends and correlations.
- Model Updating: The system updates its model based on new data and experiences to improve accuracy.
- Action Execution: Finally, the AI executes decisions while continually monitoring the results to refine its approach in the future.
Overview of Algorithms and Technologies Used
The backbone of agentic AI comprises several advanced algorithms and technologies, each contributing to the overall functionality of these systems:
- Machine Learning: Fundamental for allowing AI to learn from its data over time, enhancing its accuracy and efficiency.
- Neural Networks: Used for enabling deep learning, particularly in recognizing patterns in complex data structures.
- Natural Language Processing (NLP): Assists in understanding human language, enabling more intuitive interactions between humans and AI.
- Reinforcement Learning: A type of machine learning where AI learns to make decisions by receiving rewards or penalties based on its actions.
Comparative Analysis with Traditional AI
Unlike traditional AI systems, which rely heavily on human input for data processing and decision-making, agentic AI is designed to operate with a higher degree of autonomy. Here are key differentiators:
- Dependency on Human Oversight: Traditional AI often requires ongoing human supervision, while agentic AI can function with less human intervention.
- Capacity for Self-Improvement: Agentic AI has the ability to learn from its experiences, progressively improving its performance without needing complete reprogramming.
- Real-Time Adaptation: While traditional AI may struggle to adapt in real-time, agentic AI can swiftly adjust its actions based on new information.
- Autonomous Interaction: Traditional systems often follow pre-defined scripts; in contrast, agentic AI can take initiative in problem-solving scenarios.
Applications of Agentic Human AI
Use Cases in Business and Industry
Deployments of agentic AI span various industries. Here are some prevalent use cases:
- Customer Service: Chatbots and virtual assistants powered by agentic AI can handle customer inquiries autonomously, providing immediate assistance and recommendations.
- Supply Chain Optimization: AI systems can analyze market data and optimize logistics processes, ensuring timely delivery and cost reduction.
- Healthcare Diagnostics: Agentic AI enables diagnostic systems to assess patient data and make preliminary assessments, aiding healthcare professionals in treatment decision-making.
- Financial Services: Fraud detection systems utilize agentic AI to identify anomalous transactions in real-time, safeguarding user accounts.
Impacts on Daily Life and Work Environments
The integration of agentic AI into daily life is affecting how we work and live:
- Enhancing Productivity: By automating repetitive tasks and providing smart recommendations, employee productivity can significantly increase.
- Improved Decision-Making: Access to real-time data processing allows for more informed and faster decisions in business and personal contexts.
- Shaping User Experiences: Personalized AI-driven interfaces can adapt to individual preferences, enhancing the user’s interaction with technology.
- Facilitating Remote Work: Advanced AI tools help manage remote workplaces, enabling teams to collaborate effectively regardless of location.
Potential for Social Change
Agentic AI holds the potential to facilitate significant social transformations by:
- Democratizing Information Access: By making sophisticated AI tools available to various sectors, we can improve access to resources and information globally.
- Enhancing Education: Adaptive learning platforms can personalize educational experiences, catering to diverse learning styles and paces.
- Addressing Social Inequities: AI can analyze social data and help in formulating policies aimed at reducing inequalities in various spheres.
Challenges and Ethical Considerations
Risks of Autonomous Decision-Making
While the benefits of agentic AI are substantial, its autonomous nature also presents significant risks:
- Accountability Issues: Determining responsibility for decisions made by AI systems can be complex, raising legal and ethical dilemmas.
- Potential for Bias: If AI systems are trained on biased data, their outputs may propagate existing inequalities or create new biases.
- Reliability Concerns: An over-reliance on autonomous decisions might lead to adversities if the AI encounters scenarios it hasn’t been designed to handle.
Privacy and Security Implications
Agentic AI’s functionality often requires access to vast datasets, including personal information. This raises several privacy and security concerns:
- Data Misuse: Without stringent regulations, personal data may be misused, leading to privacy breaches.
- Cybersecurity Threats: Enhanced capabilities might attract malicious actors looking to exploit vulnerabilities in AI systems for nefarious purposes.
Frameworks for Ethical Implementation
To navigate these challenges responsibly, several frameworks and best practices for ethical AI implementation are recommended:
- Transparency: Ensure that AI systems operate in a transparent manner, facilitating understanding and trust from users.
- Accountability Measures: Establish clear lines of accountability, allowing stakeholders to address ethical concerns related to AI actions.
- Inclusive Dataset Frameworks: Promote the use of diverse and representative datasets in training AI to mitigate biases.
- Regulatory Engagement: Collaborate with policymakers to develop regulations that safeguard privacy while advancing AI applications.
The Future of Agentic Human AI
Trends and Innovations on the Horizon
Looking ahead, several trends will shape the trajectory of agentic AI, including:
- Integration of Emotional Intelligence: Future systems may be designed to recognize and respond to human emotions, enabling more empathetic interactions.
- Expansion into New Sectors: As technology matures, agentic AI will be adopted in more diverse fields, including agriculture, environmental management, and smart cities.
- Increased Focus on Sustainability: AI can facilitate solutions to global challenges, including climate change, by optimizing resource use and reducing waste.
Predictions for Workforce Integration
The integration of agentic AI into the workforce is anticipated to lead to a major transformation in how work is structured:
- Collaborative Work Models: Hybrid systems where humans and AI collaborate are expected to evolve, allowing each to leverage their unique strengths.
- Creation of New Roles: As traditional jobs transform, new roles focused on supervising, training, and working alongside AI will emerge.
- Reskilling and Upskilling: The shift towards agentic AI necessitates a significant focus on reskilling workers to thrive in an AI-driven economy.
Long-term Effects on Society
Agentic AI holds the potential to redefine societal structures. The long-term predictions include:
- Enhanced Accessibility: The reduction of barriers in technology access may lead to a more equitable society.
- Improved Quality of Life: With greater efficiencies, society can enjoy higher standards of living, benefiting from improved services and reduced costs.
- Increased Ethical Awareness: As AI becomes more embedded in our lives, there is likely to be a heightened public focus on AI ethics and its implications for human rights.