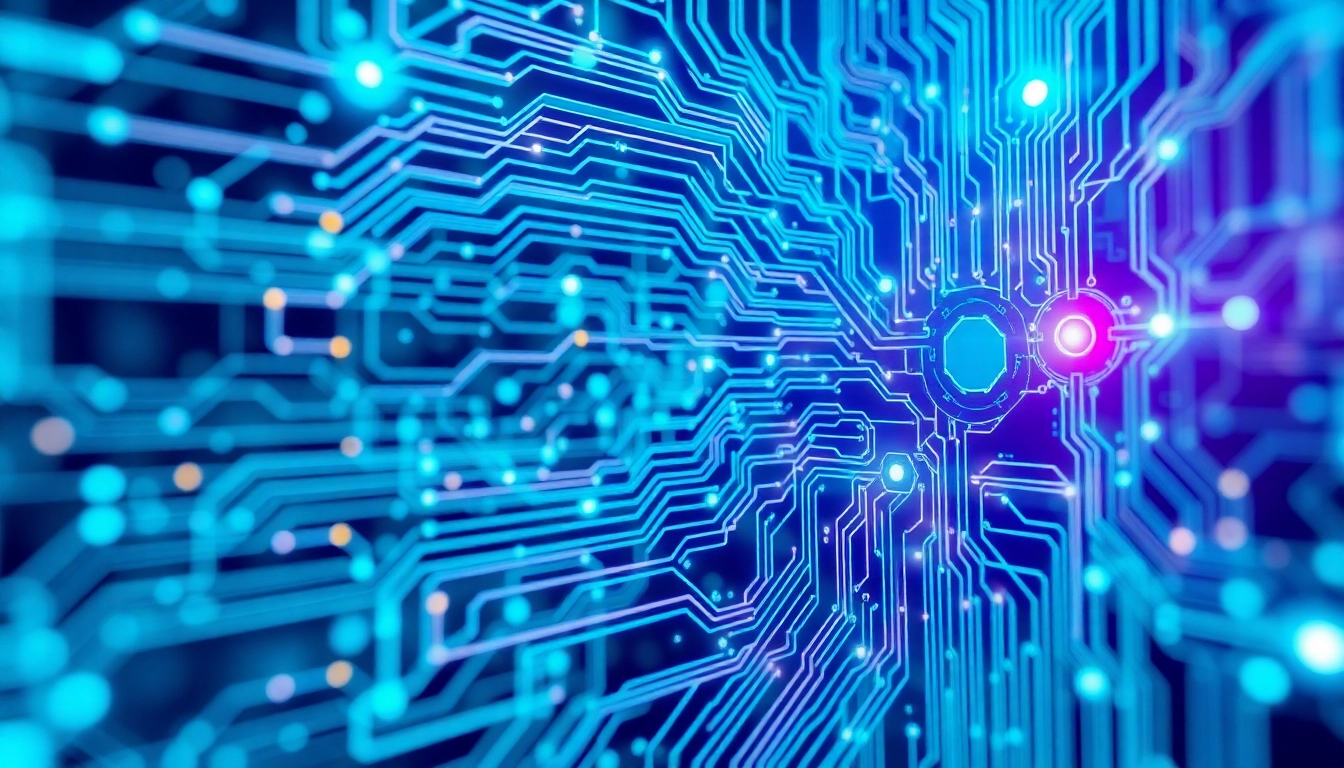
1. Introduction to Agentic AI
In recent years, the rapid evolution of artificial intelligence (AI) has shifted the way we perceive technology’s role in our lives. No longer limited to task automation, AI has grown to encompass various sophisticated systems designed to autonomously make decisions and act without constant human oversight. One of the most exciting developments in this arena is Agentic AI, a term that signifies a leap toward more intelligent, autonomous decision-making models.
1.1 Definition and Core Principles
Agentic AI refers to systems that can operate independently, learning from their environment, making decisions based on past experiences, and adapting to new challenges. Unlike traditional AI models, which typically rely on predefined rules and data inputs, agentic systems utilize sophisticated reasoning and iterative planning. They are concerned with achieving specific goals and can engage in complex, multi-step problem-solving.
1.2 Historical Context and Evolution
The concept of AI dates back to the mid-20th century, with pioneering efforts focused on rule-based intelligence systems. Over decades, we have witnessed the emergence of self-learning algorithms, most prominently through machine learning (ML) and deep learning technologies. Agentic AI represents a natural progression from previous models, utilizing advanced ML techniques to facilitate autonomous behavior, including predictive analytics and natural language processing.
1.3 Importance in Today’s Technology Landscape
In today’s tech-savvy world, the importance of Agentic AI cannot be overstated. As organizations seek ways to increase efficiency and innovation, agentic systems’ ability to handle complexity and uncertainty becomes invaluable. Industries ranging from healthcare to finance are beginning to leverage this technology to gain insights and achieve objectives that were previously unattainable through manual processes.
2. Key Characteristics of Agentic AI
2.1 Autonomy and Decision-Making Capabilities
One of the defining features of Agentic AI is its autonomy. These systems can make decisions based on the context of their environment, processing data independently of human intervention. This leads to robust, effective outcomes that agilely respond to changing conditions, enhancing operational efficiency.
2.2 Learning and Adaptation Mechanisms
Agentic AI systems are equipped with learning mechanisms that allow them to adapt to new data and increasingly complex scenarios. Using reinforcement learning, for example, these systems undergo a process of trial and error to discover the best actions for any given situation, significantly improving their performance over time.
2.3 Comparative Analysis with Generative AI
Although both Agentic AI and generative AI are crucial components of the AI ecosystem, they serve different purposes. Generative AI focuses primarily on content creation, such as writing text or generating imagery, whereas agentic AI is oriented towards outcomes and actions. This fundamental difference underscores the unique capabilities of agentic systems, as they utilize advanced decision-making processes to achieve objectives.
3. Applications of Agentic AI
3.1 Use Cases in Various Industries
Agentic AI finds applications across multiple sectors. In healthcare, for example, it can analyze patient data to suggest personalized treatment plans. In finance, agentic systems can identify fraudulent transactions in real time, providing greater security and reliability. Similarly, in supply chain management, these systems can predict disruptions and optimize logistics, leading to cost savings and improved operational resilience.
3.2 Impact on Business Operations
The implementation of Agentic AI positively impacts business operations by streamlining processes and reducing manual intervention. Companies report decreased operational costs and improved efficiency, leading to a higher return on investment. Furthermore, such systems can empower employees by freeing them from routine tasks, enabling them to focus on more strategic initiatives.
3.3 Enhancements in User Experience
In addition to operational efficiencies, Agentic AI plays a crucial role in enhancing user experience. Through personalized recommendations and customer service automation, these systems can provide tailored experiences that meet user needs swiftly. As a result, companies can foster greater customer satisfaction and loyalty, which are integral to long-term success.
4. Challenges and Limitations
4.1 Ethical Considerations
Despite its advantages, the rise of Agentic AI also raises significant ethical questions. Concerns about privacy, accountability, and the potential for biases in decision-making processes are paramount. Organizations must establish clear guidelines and ethical standards to ensure that agentic systems operate fairly and transparently.
4.2 Technical Challenges in Implementation
Implementing Agentic AI is not without challenges. Organizations face technical hurdles related to data quality, integration with existing systems, and ensuring security in operations. Moreover, the complexity of these systems can lead to difficulties in understanding and interpreting their decision-making processes, posing a barrier to widespread adoption.
4.3 Future Limitations and Research Directions
As the technology evolves, so too do its limitations. Future research will need to address scalability issues and the potential lack of interpretability in decisions made by agentic systems. As such, ongoing investigation into building more transparent models and enhancing their capabilities in understanding context will be critical for further developments in the field.
5. Future Trends and Innovations in Agentic AI
5.1 Predictive Trends for 2025 and Beyond
The ongoing advancements in Agentic AI signal promising trends for the future. By 2025, we can anticipate increased adoption across industries as organizations recognize the value of autonomous decision-making. Furthermore, as capabilities improve, agentic systems are expected to evolve into more intuitive interfaces that can better understand and anticipate human needs.
5.2 Emerging Technologies Affecting Agentic AI
Emerging technologies such as quantum computing and advanced neural networks will likely drive the capabilities of Agentic AI forward. Quantum computing holds the potential to significantly enhance processing speeds and data analysis, while advancements in neural networks can lead to more refined and effective learning algorithms, providing a substantial boost to agentic capabilities.
5.3 Concluding Thoughts on the Role of Agentic AI
As we navigate a future increasingly influenced by AI, Agentic AI stands out as a pivotal player in shaping how organizations operate. Its blend of autonomy, learning, and decision-making has the potential to revolutionize industries, providing solutions that enhance both efficiency and user satisfaction. As we continue to explore the implications and potential of this technology, a balanced approach that considers both the advantages and ethical responsibilities associated with Agentic AI will be essential to harness its full potential for society at large.