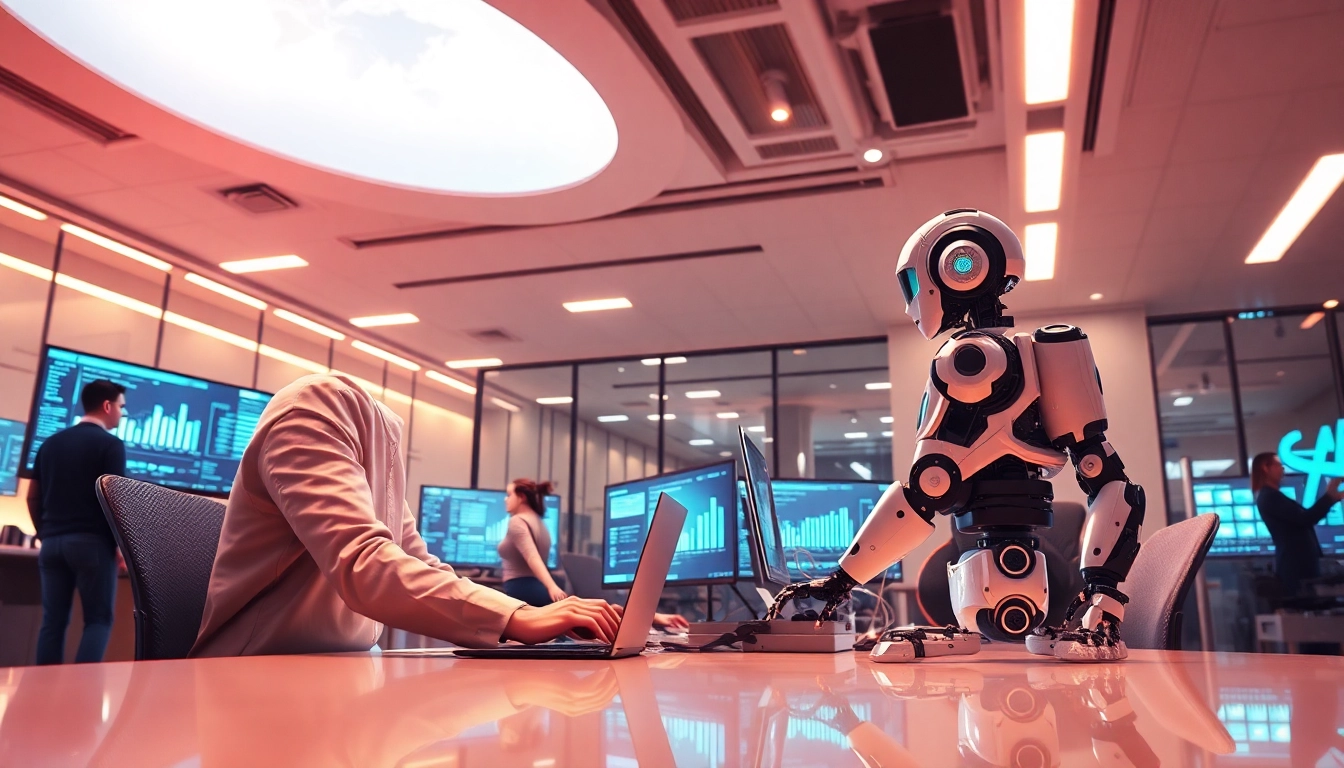
Introduction to Agentic Human AI
The rise of artificial intelligence (AI) has marked a significant turning point in human evolution, paving the way for what is now known as Agentic Human AI. This term encapsulates a category of AI that operates with a considerable degree of autonomy, capable of executing tasks without constant human intervention. This capability signifies a shift from traditional AI paradigms to systems that can act independently, make complex decisions, and adapt to changing circumstances. In this article, we will explore the intricacies of Agentic Human AI, its applications, challenges, and future prospects.
What is Agentic Human AI?
Agentic Human AI refers to AI systems designed to perform tasks autonomously to achieve specific objectives, often utilizing advanced algorithms and machine learning techniques. Unlike conventional AI systems, which typically require human oversight for every step, agentic AI can assess situations, process data, and make informed decisions. For instance, a self-driving car illustrates the capabilities of agentic AI—navigating through traffic, responding to unforeseen circumstances, and improving performance based on real-world experiences.
Historical Context and Evolution
The evolution of AI has spanned several decades, beginning with simple rule-based systems and advancing to modern machine learning and deep learning techniques. Agentic AI marks a significant leap forward from previous iterations by incorporating elements of autonomy and adaptability. Early AI systems relied heavily on predetermined rules and human input, while today’s models utilize vast datasets and sophisticated algorithms to learn from experiences. The development of machine learning, particularly reinforcement learning, has been pivotal in enabling AI to operate independently and navigate complex environments.
Key Terminology and Concepts
Understanding Agentic Human AI requires familiarity with specific key terms and concepts:
- Autonomy: The ability of an AI system to operate independently without human intervention.
- Machine Learning: A subset of AI involving algorithms that learn from data to make predictions or decisions.
- Reinforcement Learning: A type of machine learning where agents learn to make decisions by receiving rewards or penalties based on their actions.
- Natural Language Processing (NLP): The capability of a computer system to understand and respond to human languages.
Applications of Agentic Human AI
Real-World Use Cases
Agentic Human AI has found applications across various domains, transforming industries and enhancing efficiency. Some notable real-world use cases include:
- Healthcare: AI-driven diagnostic tools can analyze medical images and patient data, offering predictive insights that assist healthcare professionals in making informed decisions.
- Finance: Automated trading systems utilize agentic AI to analyze market conditions, execute trades, and optimize investment strategies with minimal human oversight.
- Transportation: Autonomous vehicles leverage agentic AI to navigate and make split-second decisions based on real-time data from their surroundings.
- Manufacturing: AI systems in production lines monitor processes and equipment, optimizing operations by predicting maintenance needs and reducing downtime.
Industry-Specific Applications
Different industries are harnessing the power of agentic AI to improve various aspects of their operations:
- Retail: Intelligent recommendation systems analyze customer behavior to personalize shopping experiences, boosting sales and customer satisfaction.
- Telecommunications: AI-driven chatbots manage customer inquiries efficiently, reducing the workload on human agents and providing instant support.
- Aerospace: Agentic AI is used in flight control systems to enhance safety and efficiency by autonomously managing aircraft operations during flight.
Benefits of Implementation
Implementing agentic AI can yield numerous benefits for organizations:
- Increased Efficiency: Agentic AI systems can process information and execute tasks faster than humans, significantly enhancing operational efficiency.
- Cost Reduction: By reducing the need for human oversight in routine tasks, businesses can lower operational costs and direct resources toward more strategic initiatives.
- Improved Decision-Making: AI systems can analyze vast amounts of data to produce insights, leading to more informed and data-driven decision-making processes.
- Scalability: Organizations can scale their operations more effectively with AI systems than with human resources alone, allowing them to respond to market changes swiftly.
Challenges and Ethical Considerations
Regulatory and Compliance Issues
As agentic Human AI continues to evolve, regulatory frameworks are struggling to keep pace. Organizations must navigate complex compliance landscapes, particularly when deploying AI systems in sensitive domains such as healthcare and finance. Ensuring that AI systems adhere to established regulations protects user data and maintains public trust. Additionally, organizations may face legal challenges if autonomous decision-making leads to unintended consequences, presenting a need for robust governance frameworks.
Balancing Autonomy with Human Oversight
While agentic AI promotes autonomy, there remains a critical need for human oversight to mitigate risks. Striking the right balance is essential, as complete reliance on AI can lead to errors and accountability issues. Effective oversight mechanisms must be established, enabling human operators to intervene when necessary. This includes developing monitoring systems that provide transparency into AI operations and decision-making processes, allowing stakeholders to understand and trust AI-generated outputs.
Risks of Over-Reliance on AI
Over-reliance on agentic AI can pose several risks, including:
- Loss of Human Skills: As organizations increasingly depend on AI, essential human skills may deteriorate due to lack of practice, potentially jeopardizing job security.
- Decision-Making Errors: AI systems are not infallible; they can make incorrect decisions based on flawed algorithms or biased data, leading to negative outcomes.
- Ethical Concerns: The use of autonomous systems raises ethical dilemmas regarding accountability and bias, particularly if AI systems are making decisions that impact individuals’ lives.
Future Trends in Agentic Human AI
Emerging Technologies
The development of agentic AI will be characterized by a few emerging technologies poised to further enhance its capabilities. Some key trends include:
- Deep Learning Advancements: As deep learning techniques evolve, agentic AI will be capable of processing and analyzing more complex datasets, resulting in more accurate and nuanced decision-making.
- Explainable AI (XAI): Enhancing the transparency and interpretability of AI decisions is critical. Efforts toward develop XAI will help stakeholders understand how and why AI systems arrive at specific conclusions or actions.
- Quantum Computing: The advent of quantum computing may revolutionize AI by enabling faster computational speeds and more complex problem-solving capabilities, significantly boosting the efficacy of agentic systems.
Impact on Workforce Dynamics
Agentic AI will significantly alter workforce dynamics. While it promises increased efficiency and productivity, it may also require shifts in workforce skills and roles. Organizations will need to prepare for a future where human workers collaborate with AI to achieve common goals. This new landscape will necessitate training and upskilling programs to equip employees with the skills required to work alongside AI systems effectively.
Predictions for Advancements
As the arena for agentic AI expands, significant advancements are on the horizon:
- Widespread Adoption: More organizations across industries will adopt agentic AI solutions, recognizing the competitive advantage they offer.
- Enhanced Interoperability: Future agentic AI systems may better integrate with existing technologies and platforms, promoting seamless collaboration between AI and human workers.
- Focus on Ethical AI: Greater emphasis will be placed on developing ethical frameworks for AI deployment, including fairness, accountability, and transparency.
Strategies for Successful Integration
Creating an AI-Ready Culture
To successfully integrate agentic AI, organizations must foster an AI-ready culture. This involves:
- Encouraging open dialogue about AI’s role in the workplace and its potential benefits and challenges.
- Promoting a mindset of adaptability and embracing change among employees.
- Ensuring that leadership actively supports and invests in AI initiatives, signaling their importance to the organization’s success.
Training and Upskilling Employees
Investing in employee training is critical for maximizing the benefits of agentic AI. Organizations should implement comprehensive upskilling programs that focus on:
- Equipping employees with foundational AI knowledge to foster a better understanding of how AI systems operate.
- Providing practical training on collaborating with AI tools, focusing on the synergistic potential between human workers and AI.
- Encouraging continuous learning to ensure employees can adapt to evolving AI technologies and methodologies.
Monitoring Performance and Impact
To ensure successful integration, organizations must establish metrics and systems for monitoring the performance and impact of agentic AI initiatives. This involves:
- Setting clear KPIs to assess how well AI systems achieve desired outcomes.
- Implementing feedback loops that allow organizations to make adjustments and improvements based on real-world performance.
- Engaging in continuous evaluation processes that analyze the ramifications of AI deployment on workplace dynamics and culture.